Machine translation and generative AI (part 2): disadvantages
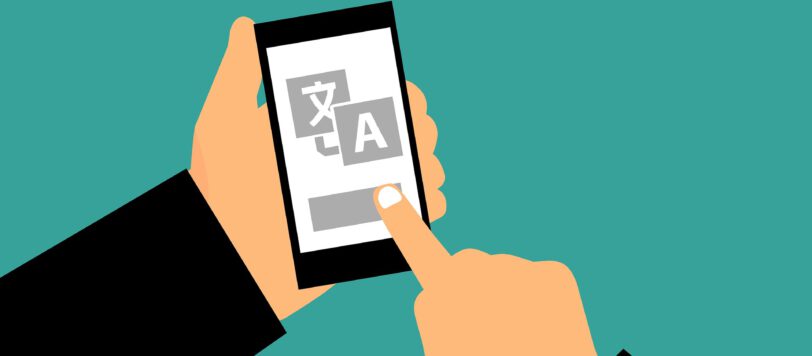
The disadvantages of machine translation
In my February post I gave you a short introduction to machine translation and generative AI (Artificial Intelligence) in translation. And I talked a bit about their distinct advantages since they have by now become important tools in our ever more connected world.
In this month’s post, I would like to take a closer look at the disadvantages of machine translation. You may now say that as a trained (human) translator I might have a bit of a bias, but the point of this post is not to bad-mouth this technology—it is rather to draw attention to all the little things that are not mentioned in their marketing or might not be known by the general public.
(Interesting little fact for you: the inspiration for this post was this article: Amazon Researchers Find ‘Shocking Amount’ of Faulty Machine Translations in the Web. Here, an Amazon AI research group reports being worried about the quality of machine translation. The reason is that a lot of texts on the web are now AI generated texts and/or machine translated materials of sometimes shockingly bad quality, but these are being used as training data for new translation engines, which has a negative impact on their output quality. I definitely recommend reading it!)
Issues arising from automated translations
Machine translation actually still has a surprising amount of issues it has to contend with. In order to make following them a bit easier, I have grouped them in three categories: the translation of texts, the translation of speech (“machine interpreting”) and general problems (with two sub-categories, language and technology).
Translation of texts
Accuracy: the devil is in the details
Even as a trained translator, I am more than happy to admit that the quality of machine translation has greatly increased in the last few years. Especially the advent of neural networks and natural language processing has led to sometimes very good translations, at least in the “big” languages such as English, German, French or Spanish (which are also closely related). But this big increase in quality also poses a risk of its own: this, at first sight, very good output makes it more likely that we tend to overlook smaller mistranslations and shifts in tone because we trust the machine and its output. But these little mistakes and shifts can potentially change the meaning or feel of a text radically! This is especially dangerous in cases where the user of the machine translation tool does not speak the language into which a text was translated fluently or at least to a very high level: wrong nuances or erroneous translations are then just copied from the tool in good faith and used as if they were correct.
Typos
As of yet, most texts are still written by humans. And it is no secret that humans make mistakes, especially when it comes to grammar and spelling. Modern spell and grammar checks are very good, but they cannot pick up everything—especially when the typo ends up being a correct word! In the worst case, such typos can change the entire meaning of a sentence. And a machine does not question this, it only translates. But a human translator would.
No holistic view
Machine translations tend to process texts in subunits, which are usually single sentences. This means that the output is generated sentence by sentence, sometimes leading to strange mistakes: for example, it happens relatively frequently that the same word would be translated in three different ways in three subsequent sentences, because the machine deemed each word as the most plausible solution for the sentence in question. This can be a great issue inhibiting readability and consistency and could potentially lead to confusion.
Translation of speech
Speech to text—translation—text to speech
Even the process of machine interpreting in itself is tricky. Current machine interpreting tools are unable to directly translate speech directly; instead, they first transform speech to text with the help of speech recognition, then they translated this text into the other language (just like in normal text translation) and in the last step, they generate speech from this text. Especially the first step of this process can be quite error-prone (as we can see when using automatically generated closed caption on YouTube for example), because the machine does not always understand us correctly. And if the text base for the translation is already wrong, the machine translation will be incorrect as well.
(Note: this does not necessarily mean that communication will break down, but it would be an issue in situations where nuances are key—such as business negotiations.)
Accents and dialects
Not every speaker of English speaks “standard English”. (Which also opens up the question to what that would be in the first place—Oxford English? Certain variations of American, Canadian, Australian, South African English? Or something entirely different?) As great as regional variation is, it can be very problematic for machines: strong accents and dialects might lead to the speech recognition step failing in part or even completely, which would make a translation impossible.
Modulation
Speech is much more than just words. We all know that the tone of voice and its modulation carry meaning as well: they tell us how the other person feels about the topic, we use them to stress certain information and to communicate what we think about what is being said. Human interpreters try to emulate this, but machines cannot—certainly not to date. Partly, because they work based on text. But this leads to a loss of information that can be crucial to communication.
Flow of a conversation
The flow of a conversation and the “turn-taking” of the different speakers can also be problematic for machine interpretation tools, especially in cases where the technology is used by one person who does not speak the language everyone else in the room shares. This larger group might end up interrupting each other or trying to override other speakers to make their point. But the machine does not distinguish between different speakers, it might not understand everyone clearly and could therefore end up delivering a confusing or disjointed interpretation.
Misspeaking
This is again about us as humans making mistakes. Misspeaking is a bit like the typos of spoken language: it can lead to confusing translations and changes in meaning, which could in turn lead to misunderstandings. Human interpreters would check back to clarify, but a machine would not.
General problems
Technical aspects:
Bias of training data
In order to produce high-quality output, machine translation systems have to be trained with vast amounts of data. This data, however, unfortunately often contains biases introduced by the various authors, which in turn means that the machine learns certain prejudices which it cannot question or put in a certain context because it lacks the capability to do so. One interesting example is that in certain languages, statements expressed in a feminine form are interpreted as more negative than the same statements in masculine form .
This is also a problem in generative AI. In many cases, prompts phrased in masculine form deliver more successful results than the same prompt phrased in a feminine form (as heard in the German-language podcast „KI verstehen“ (“Understanding AI”) by Deutschlandfunk).
Data privacy
One of the most important issues with modern machine translation tools (which many people are unaware of) is the privacy and protection of sensitive data. Most providers’ terms and conditions state that the data put in by the user will be used for further training purposes. (There are some exceptions when using paid plans though.) If employees who are unaware of this enter sensitive company information, they pass on proprietary and potentially secret information to the provider of the respective machine translation tool.
Computational power and internet connection
As mentioned before, modern machine translation tools work with a technology called “neural networks.” These are highly complex systems which need a lot of computational power and training, which is why most users access them via the internet. But this also means that if the internet fails because of a power cut or an interrupted connection, there will not be any translations or interpretations (which is a problem in important meetings or negotiations, for example).
Language-related aspects:
Terminology and consistency
If you ever had a peek inside a two-language dictionary, you know that only very few words have only one corresponding translation. Most of the time, there are multiple solutions and which one is correct depends on context. The vast amounts of training data machine translation tools need means that the tool learns a lot of possible translations and contexts. It is therefore difficult to “force” them to use a certain translation for a certain word. Especially in highly technical contexts, the tool might not even have come across certain technical terms in its training data. And, as mentioned in the “No holistic view” section above, it is not uncommon for such tools to translate the same term in various different ways across the text.
Along with technical terminology, names also pose a problem: if they are newly invented, obviously “artificial” words, machine translation tends to recognise this and just leaves it as is. But if it could be a legitimate name or word from everyday language, translation tools just translate them without questioning whether this would be wanted or make sense.
Grammar
Most machine translation tools nowadays produce very good grammar output and fluent sentences. But grammar is still a major source for errors: German, for example, has two-part verbs where the second part of the verb can be at the end of the sentence and first part close to the beginning. Sometimes, the second part at the end of the sentence is then omitted by the machine translation, making it a confusing sentence.
Another good example for grammar interference is Polish: this language works with verbs that are gendered masculine and feminine. If translating from a non-gendered language such as English, there is a high risk of picking the wrong gender for the intended audience and this way putting them off.
The human factor
The thing that really makes human communication difficult for machines its inherently human nature. Irony and sarcasm play an important role, but when translated by a machine, the tone of voice is lost completely, which could lead to such a tongue-in-cheek statement being taken seriously.
Puns and idioms also pose a lot of difficulty. Especially puns do not tend to make any sense after translation and the intended laugh and defusing of a potentially tense situation therefore will not happen. Ambiguous statements, which could be cleared up by a human interpreter, are also problematic for users of machine interpretation.
Another very human aspect is phrasing statements differently depending on who we are addressing. Machines do not know who they are talking to and therefore do not distinguish between different audiences or reasons for communication. They just follow their programming. An understanding of different cultural concepts (such as conventions when addressing someone) is also difficult to implement in machine translation tools, whereas human translators and interpreters would be aware of these.
Summary
Congratulations if you made it to the end of this very long post! I have to admit that when I started collecting material for this topic, I had not expected it to be this extensive a text…
But this shows us again how many aspects of daily communication happen subconsciously. And the subconscious does not tend to draw attention to itself. I therefore found it important to highlight potential sources of errors and risks in the use of machine translation that many users might not be aware of.
This does in no way mean that machine translation and generative AI do not have a rightful place in our modern world. On the contrary! This is why in my next post, I would like to explore when machine translation is a useful or even valid tool to use. I look forward to welcoming you back!
Comments are closed.